In today's competitive, data-driven landscape, tapping into accurate and timely data is crucial for making well-informed business decisions and staying ahead of the curve.
However, two-thirds of CMOs admit to feeling overwhelmed by the sheer volume of data available to them.
One major challenge lies in the lack of a single source of truth, with people having to log into multiple systems and platforms to access and gather data, which is often presented in different formats and structures - making consistent and meaningful analysis almost impossible.
ETL automation tools can solve this issue for a lot of businesses - offering a single, standardized ‘source of truth’ that can be leveraged for data insights across the business.
What is ETL?
ETL stands for ‘extract, transform and load’, and essentially describes a three-step process of extracting data from a source, transforming it so it is cleaned, harmonized, and can be compared with other data sources, and then loading it into a target database such as a data warehouse or BI tool.
ETL components
Data extraction
Data extraction is the first stage in the ETL process, and the most critical step of the three.
Data extraction is the process of obtaining data from a particular data source. In order for ETL to be done effectively, data needs to be collected directly from its source in its rawest form.
At a basic level, an example of data extraction can be the simple act of manually exporting CSV files directly from Google Ads.
However, while this might sound simple enough, things can get much more complicated when you're looking at multiple data sources that you want to compare - which is where data transformation comes in.
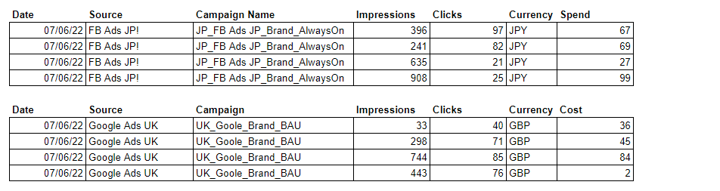
Data transformation
Transforming data means stripping it of the formatting that is specific to each data source, and applying a new set of consistent formatting across all your data sources so they can be easily compared.
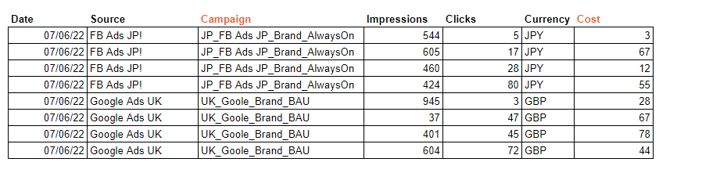
For instance, Google Ads uses the term ‘Cost’ whereas Facebook Ads uses the term ‘Spend’. For a business to draw meaningful comparisons from the two data sets, it’s necessary to reformat both sets of data so they both use the same terminology. This is commonly referred to as schema mapping, and it's a critical part of the data transformation process.
During data transformation, it’s also possible for data to be enriched - for example, by converting everything to a single currency or adding additional region and channel information.
Some ETL processes will also include a naming convention standardization phase during data transformation, to ensure that things like campaign names are all consistent and any errors are corrected.
Data loading
The final step in the ETL process is data loading, where transformed data is loaded into a target destination for analysis, such as business intelligence (BI) tools and database applications.
At this stage, marketers and marketing analysts have what is sometimes called a single source of truth.
Data is now all in one place and consistent in terms of format, so teams can easily compare it and draw useful insights across different channels and platforms - enabling them to adjust their strategies and budgets accordingly.
To help illustrate how ETL can be useful in a real-world business setting, visit our case studies for Liberty International Tourism Group and Cosmonauts & Kings.
ETL vs ELT
ETL is a well-established process of data integration that helps businesses get all their data harmonized and ready for analysis.
But it’s not the only way that businesses can provide data analysts and marketing teams with a single source of truth to deliver meaningful insights.
Another data integration approach is ELT, which stands for “extract, load and transform”.
ELT has all the same stages as ETL, just in a different order. Rather than transforming the raw data before loading to a database or data warehouse, ELT loads the raw data into the target destination first, with any cleaning and harmonization being completed by the data warehouse.
ETL and ELT are often discussed as competing ‘either/or’ approaches, but it’s actually more useful to view them as complementary as they both have different use cases.
If businesses have a clearly defined way they want to view certain structured data, or have limitations on the processing capabilities of their data warehouse or BI tool - cleaning and harmonizing that data before it’s loaded with ETL might be the best solution.
On the other hand, for some data sources, businesses might be able to gain additional valuable insights by allowing teams the freedom to analyze and experiment with raw data, which makes ELT a more suitable approach.
It all depends on specific business needs. With an integrated data platform, businesses can easily benefit from the advantages of both ETL and ELT processes.
Data extraction methods
Small businesses with only a handful of data sources might consider performing the ETL process manually. While this might seem feasible in the short term, it can be labor intensive and can be detrimental to the motivation of the specialist completing the repetitive work. Manual handling of data can also increase the likelihood of inaccuracies because of human error, and can be problematic for data governance.
On the other hand, if you’re a larger organization with a few dozen different data sources that you want to integrate - automated ETL tools are a necessity.
Before we run through the features that you might want to look for when choosing an ETL tool for your business, let’s look at the three different ways that they can pull in data.
Full extraction
Full extraction is typically used when setting up new databases or business intelligence tools.
As the name suggests, this method extracts and transfers the full source dataset, transforms it, and then sends it to the data destination.
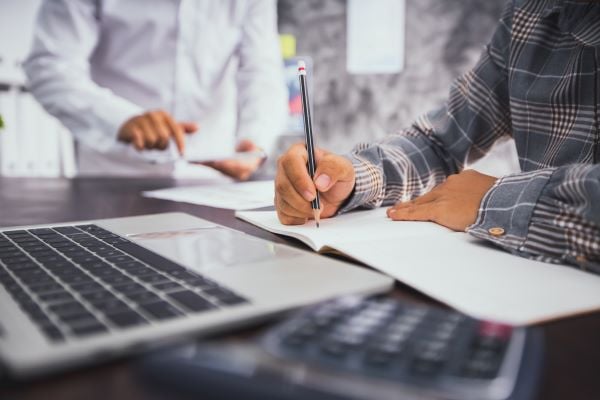
Sometimes, the volume of data to be extracted in a full extraction can be significant, for example, if your business has been collecting data within the source for several years.
It might be impractical to try to extract a huge dataset all at once - not only can it take a long time, but it also increases the likelihood of hardware failures or network errors during the transfer process which can leave you with an incomplete destination dataset.
In these circumstances, batch extraction can be an effective alternative. It essentially achieves the same goal as full extraction - but it breaks down the source data into smaller, manageable chunks (or batches), which are then extracted and transferred to the target system sequentially.
By splitting the extraction process into batches, businesses can reduce the strain on their systems and minimize the risk of errors or disruptions during the data transfer process.
Incremental extraction
Incremental extraction is the process of extracting and processing only the data that has changed or been updated since the last extraction, rather than the full dataset.
An example might be a marketing team who wants to monitor the ongoing engagement of Facebook Ads. They run an incremental extraction process at 11 PM each day - extracting, transforming and loading the new data from the last 24 hours.
This incremental approach is far more efficient than bringing in large sets of data at less frequent intervals. But perhaps the major benefit is that incremental extraction ensures that teams within the business can make strategic optimizations based on accurate, up-to-date information.
Source-driven extraction / Change data capture
Source-driven extraction refers to the approach in which the extraction of data is driven by changes in the source data, rather than by a pre-set schedule.
In other words, data is extracted, transformed, and transferred to the target data destination as soon as it changes in the source system.
The aim of source-driven data extraction is to ensure that the most recent data changes are immediately available in the target data destination - which is why it's often seen in real-time data integration.
Change Data Capture (CDC) is a method of source-driven data extraction. With CDC, when data in the source is created, changed, or deleted, those changes are captured and made available to be extracted, transformed, and loaded into the target destination.
What is ETL in data integration?
While the terms ETL and data integration may sometimes be used interchangeably, it is important to note that ETL is actually a subset of data integration, specifically dealing with the extraction, transformation, and loading of structured data.
Data integration on the other hand includes a wider array of techniques, encompassing ETL, ELT (Extract, Load, Transform), data federation, and data virtualization.
The reason why ETL is sometimes referred to as ‘data integration’ is because it’s arguably the most common way that businesses choose to integrate data, as structured data (data arranged in rows and columns, and stored in a relational database) powers the majority of businesses' performance analysis and reporting.
Data integration solutions like Adverity often include comprehensive ETL functionality to support data teams within businesses, such as hundreds of pre-built connectors to connect to various data sources, the ability to transform and enrich data, and the option to load data into any destination for analysis.
ETL automation tools
If you’re looking to unlock the power of your data, and improve the accuracy, timeliness and data governance of your business information - you should be using an ETL automation tool.
By embracing solutions that automate the ETL process, your business can access a wide range of benefits over trying to manually integrate data:
Save business time and resources
ETL automation tools can significantly reduce the time spent on manual data processing tasks. They're able to efficiently extract data from multiple sources, transform and enrich it according to your business needs, and load it into the destination system without the need for human intervention.
Reduce costs
Automated ETL processes also eliminate the need for hiring additional resources to manage manual data integration tasks. This can help to reduce overall business costs and allow data teams to focus on more strategic insight-driven activities that can help business performance.
Improved data accuracy
Manual ETL processes can be prone to human error, potentially leading to inaccurate data. ETL automation tools ensure that data is processed consistently and accurately, minimizing errors and improving overall data quality.
Enhanced security
Most ETL automation tools have advanced security and data governance features designed to protect your sensitive data during the integration process and allow you full control over things like data access and data retention.
The ability to scale
With ETL automation tools, businesses can effortlessly scale their data integration efforts as their data volume and complexity increases.
If you’ve decided that an automated ETL solution is the right choice for your business, you’re going to feel spoilt for choice. There are a lot of different solutions on the market.
You’ll possibly want to evaluate your options based on six main criteria:
Connections
The majority of ETL solutions have a library of pre-built connectors that allows data teams to quickly and easily extract data from popular data sources. The number of pre-built connectors varies by solution - some might only have 70, whereas others boast more than 600.
It’s also worth asking if each of the solutions you’re considering supports the ability to code your own custom connectors, so you can extract all of your bespoke business data.
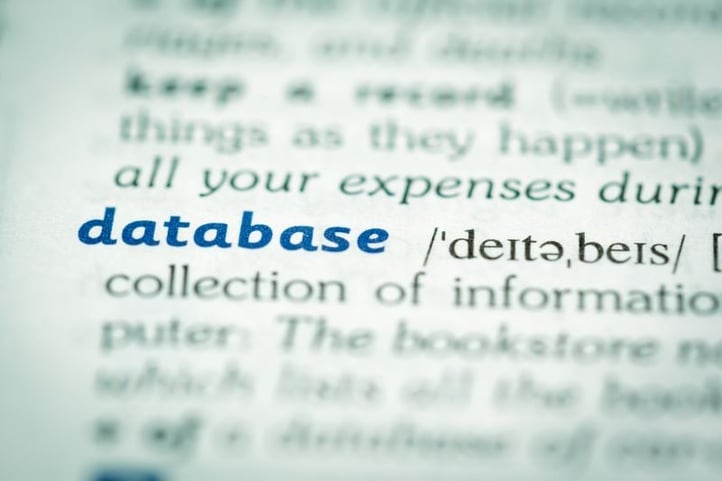
Transformation capabilities
You’ll also want to consider the data transformation and enrichment capabilities of different ETL solutions.
Most ETL automation tools will offer standard features such as schema mapping, but some are far better in the arena of data enrichment if your business is looking to enhance the value of the data being extracted.
Support for different data destinations
You might also want to query the different data destinations that ETL tools are able to load your data to, as some solutions are more flexible than others. To future proof your integration, you ideally want to be choosing a solution that can send your data to any destination you want, whether it’s cloud storage, a data warehouse, data lake or BI tool.
Data management
It’s important to query the measures that each solution has in place to help you maintain accurate data.
Some solutions have in-built functionality that helps to enforce naming conventions, even if there are inconsistencies in source data. There are also solutions that monitor and detect anomalies in data when any of your key metrics fall outside of the expected range.
You might also want to consider how frequently each solution can extract data from your various data sources, as this is another factor that can vary by solution.
Data governance and access
It’s important that you choose a solution that has robust security and data governance capabilities to make sure that any data processing is in line with regulations, and to control things like who can access certain sensitive data and how long data is retained for.
Analysis capabilities
Some ETL tools just focus on the extract, load and transform process - and there’s nothing wrong with that.
But some automated data integration platforms that offer ETL as part of their solution go a step or two further, and actually have some in-built data analysis and visualization tools that can help businesses get the most out of their data.
Finding the best data integration platform for your business
Every business is unique, with distinct data needs and challenges that require tailored solutions.
We’ve already looked at the core functionality that you should be looking at when choosing your automated ETL solutions.
But the decision goes beyond the different functionality that each platform has. You need to make sure it’s suitable for the specific needs of your business.
Some of the key factors to consider when you’re choosing an ETL platform that aligns with your specific requirements include:
Your current and future data needs
When selecting an ETL platform, it's essential to consider both your current and future data needs.
It’s easy to get caught up in thinking about what is most suitable for you right now - but you also need to make sure the platform is future-proofed and able to accommodate your evolving business needs as your data sources and use cases for data expand.
Your internal technical capabilities
Another important factor to consider is your organization's technical capacity.
Some enterprise-level ETL tools come with a steep learning curve, requiring specialized skills and knowledge to get the most out of them. Think about whether your team has the necessary expertise in-house, or if a more user-friendly ETL solution would be preferable.
You might also want to consider the availability and quality of training guides and customer support to help you when your team has questions or queries.
Budget constraints and pricing models
Different ETL tools can vary significantly in cost, and it's essential to find a solution that aligns with your budget. It’s worth comparing the pricing models of various tools and weighing them up against the features and benefits they offer.
Compatibility with your existing infrastructure
Seamless integration with your existing data sources and data destinations is crucial for an efficient ETL process. Make sure the ETL platform you choose is compatible with your current infrastructure, allowing for smooth data integration.
Request a Demo or Trial
Before committing to an ETL tool, always ask for a demo or trial to thoroughly test its functionality, usability, and performance.
Getting this hands-on experience with the platform is the only way to effectively evaluate how well it meets your specific business needs and identify any potential compatibility issues or other limitations.
Ease your ETL process with Adverity
We've covered a lot of information in this article to help you better understand what ETL is and how to evaluate the tools that can assist you with an automated ETL process.
Now, it's time to introduce you to Adverity and its many benefits.
Adverity is a powerful data integration platform designed with a number of impressive features to simplify and streamline your ETL processes:
- More than 600 pre-built connectors to easily extract data from popular sources
The ability to create your own custom connectors. - The ability to extract data up to four times per hour from your target sources, providing your business with near real-time insights.
- Seamless data transformation processes, enabling you to combine data from all your data sources.
- Data enrichment, allowing you to convert currencies, dates and languages in your data.
- Robust data governance capabilities.
- In-built functionality to help maintain the quality and accuracy of your data, such as Smart Naming Conventions and anomaly detection.
- The ability to load your data into any data destination.
- Flexible pricing plans, suitable for businesses of all sizes
If you want to find out more about Adverity, we have a wide range of educational resources that we hope will answer a lot of your questions.
Or if you’d like to get a more interactive overview of how Adverity works, book a demo with our friendly team.