Currently, more than half of CMOs say they’re using 14 or more data sources — and more than two-thirds of CMOs (67%) say the volume of data available to them has become increasingly overwhelming. As the volume and variety of data available to marketers and data analysts increases, the importance of a streamlined solution to unify all this information into an analytics-ready data set continues to grow. This is where data mapping works its magic.
In this blog, we’ll explain what data mapping is, why it’s so important, and what benefits marketing teams can expect to see from streamlining their data dictionary. Read on to find out more, or watch out short explainer video below.
What is data mapping?
In simple terms, data mapping is the process of cleaning and combining data. It involves the reorganization of data based on a data dictionary, consisting of a predefined set of rules known as the data schema, which dictates aspects such as standardized table names, field names, and what values can be included in a field (i.e. is it a number, a date, text, etc.)
Data mapping is crucial because it creates consistency and accuracy within your data, while also saving analysts a lot of time by providing a unified language for field names across different sources, making data analysis easier and more efficient.
What does that mean?
Consider the metric "cost" - a fundamental metric for even the most basic reports. You’ll want to be able to easily compare this metric across all of your data sources. However, this key metric is called “Spend” in Facebook Ads, in Google Analytics is called “ga:adCost” and in Google Ads is called “Cost”. That’s already three different names across some of the most popular data sources — and as the list of data sources increases, so does this list of disparities.
This inconsistency creates a huge hurdle during analysis, requiring translation and alignment of field names before you can get on with basic calculations like cost per click. By data mapping these different terms to one standard term, marketers can eliminate this problem. For instance, in the above example, you could data map all those different field names to “cost”, allowing you to get on with analyzing your data across different channels.
And as an added bonus, data mapping is a perfect way to tidy up those non-user-friendly field names. Say goodbye to long field names from Facebook Ads such as “actions.onsite_conversion.post_save”, and say hello to the clean, easy-to-read “post_save”.
Why should marketers care about their data mapping?
The end goal of data mapping is to get all the data you’ve exported from different platforms in one place, in the right format, and filtered down to what you’re actually going to use so it’s ready to be analyzed. This way it’s ready to be explored and analyzed with no manual data cleaning.
By implementing pre-defined data mapping, you can seamlessly transform data into the format you need so that you can compare apples to apples. Streamlining this process means you can get right into using your data to spot risks and opportunities much more easily, and ramp up or dial down spend accordingly.
Where in the data pipeline does data mapping happen?
Data mapping can happen before or after data ingestion. Or in other words, once all your data has been fetched from the platforms you’re using.
Some companies might prefer to retain raw data and load that to a destination prior to transforming, as this allows for more flexibility in data analysis - this is known as ELT (Extract, Load, Transform).
Others prefer to apply data transformations before loading to their preferred destination to ensure data is being used in a consistent way across their organization - this is known as ETL (Extract, Transform, Load).
You can read more about the difference between ELT and ETL in our blog ETL vs. ELT: Why They’re Complementary Data Integration Solutions.
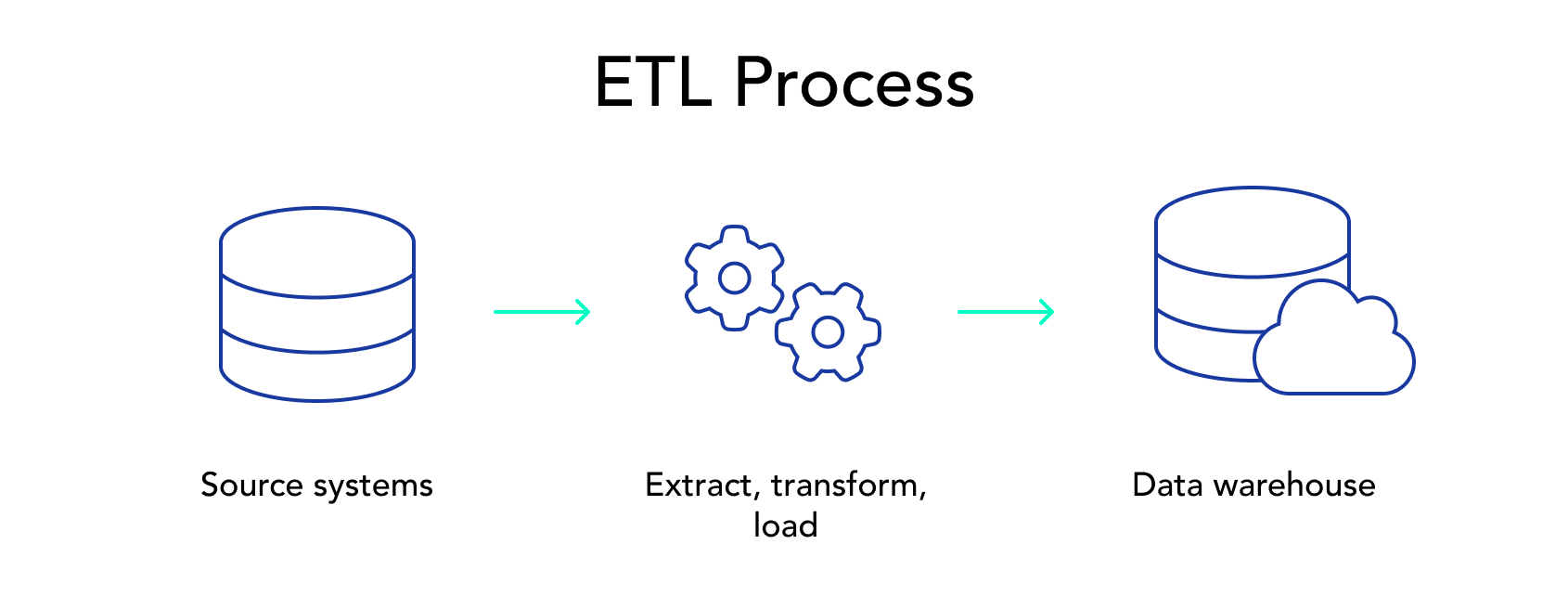
Once the data dictionary has been implemented and all the fields are mapped and unified, the final dataset can go on to be used for analysis and data modeling to figure out how to optimize activities.
What is data mapping vs. a data schema vs. a data dictionary?
In simple terms, data mapping is the name for the process of unifying data into the format you want, while a data dictionary and data schema are the blueprints for this process.
As part of the data mapping process, you can also define which fields you want to include or filter out of your final data set. So, if there’s data that you’ve fetched from your marketing platforms that you don’t want to be pushed to your chosen destination for reporting, then you can simply refrain from data mapping it and it will not clutter up your dataset.
Part of the blueprint for this process within data transformation is the data dictionary. A data dictionary is a list of ideal, front-facing field names to be used across multiple data sources.
The data schema sits behind this as the structure for which these field names are used, including information such as whether a field is a String or a Long, if it is aggregatable, and if it has any restrictions on character length. This also encompasses the metrics layer, which dictates standardized formulas for calculated KPIs such as CTR.
So in short, data mapping is the process of implementing the data transformations outlined by the data dictionary, which is powered by the data schema and the metrics layer.
For more on data dictionaries, check out our blog: How to Create a Data Dictionary in 5 Steps.
What are the benefits of streamlining data mapping?
Streamlining data mapping offers several advantages that can greatly benefit your data management and analysis processes. Here are the key benefits:
Increase efficiency and save time
By implementing standardized data mapping through a well-maintained data dictionary, you eliminate the need to rename fields each time you use them. This saves a significant amount of time and enhances overall efficiency. With a consistent naming convention, the data dictionary becomes intuitive and easily understandable to users of all levels of experience.
Create a single source of truth
Standardizing the format of your data early in the pipeline means everyone has access to the same data — this might sound small but when different teams have minor variations in how they calculate KPIs like budget, it can cause big problems for the C-suite. Mapping your data into a unified format through a data dictionary makes sure that stakeholders across your company are singing from the same hymn sheet.
Increase data accessibility across the organization
More commonly known as data democratization. A streamlined data mapping process simplifies data comprehension and accessibility. Instead of needing extensive training on various terminologies, users can focus on learning a single language, so everyone can make data-driven decisions regardless of how data-literate they are.
Avoid confusion over ownership and management
When multiple people are responsible for copying and pasting data into a spreadsheet, it’s easy to get wires crossed and break the format. By defining who is responsible for maintaining and updating the single data dictionary for your data, and automating these processes where possible, teams can ensure clear ownership and management of the data mapping, maintaining data quality.
Check out our agency guide to data storytelling!
Automating your data mapping
While data mapping can technically be carried out manually, the increasing amount of platforms and granularity of data they provide is forcing this practice into extinction. Tools to automate data integration are becoming more popular as a way to give marketers back some of their time to focus on executing data-driven decisions.
By automating data mapping, marketing teams can avoid the time-consuming and error-prone practice of copying and pasting data into spreadsheets, and create a much more scalable single source of truth that everybody can work from.