The line between valuable marketing insights and wasted effort often hinges on two key concepts: data quality and data governance.
It’s easy to lump these terms together or assume they’re interchangeable, but that’s a mistake. Let’s set the record straight and dive into what each of these terms really means, and why they’re both crucial for anyone serious about making data-driven marketing decisions.
What is data quality?
Data quality is all about the condition of your marketing data. Think of it as the health check for your data assets—are they accurate, complete, consistent, unique, timely, and valid?
When your data is of high quality, it means you can trust it to make informed decisions, drive effective marketing campaigns, and uncover valuable insights.
On the flip side, poor data quality can lead to costly mistakes, like sending marketing emails to outdated addresses or making strategic decisions based on incorrect information.
In essence, data quality is the gold standard for your data’s fitness for use. It’s what ensures that the data you’re working with is good enough to rely on.
What are the six dimensions of data quality?
There are six key dimensions to data quality that we briefly touched on in the definition, but let’s take a closer look at what these all mean and why they’re important.
Data accuracy:
Data accuracy refers to how closely data reflects the true values or the verified source of information. Accurate data correctly represents the real-world conditions or facts it is intended to model.
Ensuring data accuracy is crucial because it impacts decision-making, forecasting, and operational processes. Inaccurate data can lead to flawed decisions and decreased trust in data systems.
Data completeness:
This dimension assesses whether all necessary data is present in a dataset. Data completeness involves having no missing values or data records that are expected to be in a dataset.
Complete data is vital for performing comprehensive analysis, reporting, and business intelligence. Missing data can lead to biased conclusions and inadequate insights.
Data consistency:
Data consistency refers to the absence of difference when comparing two or more data elements or datasets that are supposed to be the same. This includes uniform formats and synchronized information across data stores.
Consistent data helps maintain reliability and accuracy across diverse systems and processes, which is essential for seamless operations and unified reporting.
Data uniqueness:
Date uniqueness means that no data records are duplicated in a dataset. Each entry is distinct and represents a unique piece of information.
Unique data prevents redundancy, ensures efficient use of storage, and supports accurate analysis. It is particularly critical in databases where primary keys require uniqueness to identify records uniquely.
Data timeliness:
Data timeliness refers to data being up-to-date and available when needed. It involves having data ready and accurate as of the required point in time.
Timely data is crucial for real-time decision-making and operational effectiveness. Delays in data availability can hinder responsiveness and lead to missed opportunities.
Data validation:
Data validation involves checking data for accuracy and cleanliness before it is used or processed. This can include format checks, rule compliance, and verification against known standards or reference datasets.
Validated data ensures that datasets are accurate and fit for their intended use, reducing errors and enhancing the reliability of data-driven decisions.
Each of these dimensions plays a critical role in ensuring that data is reliable and actionable, supporting various business processes and decision-making needs.
What is data governance?
Data governance is the framework that ensures your data is managed in a structured, secure, and standardized way across the entire organization. It’s the set of rules and guidelines that determine how data is accessed, handled, and protected.
Data governance encompasses a range of processes and roles aimed at maintaining data quality, security, and compliance. With a solid data governance strategy, you’re not just setting the stage for data quality—you’re also ensuring compliance with regulations, enhancing data security, and making data accessible to those who need it, when they need it.
In other words, data governance is the blueprint that makes sure everything about your data, including its quality, stays on track.
Key components include:
Foundational governance:
- Security: Implementing measures to protect data from unauthorized access and breaches and adhering to regulations like GDPR.
- Access and data ownership: Establishing clear documentation of who owns and has access to data within platforms, including managing permissions and creating processes to govern access.
Structural governance:
- Classification: Organizing data through systematic classification and using data dictionaries to ensure consistency and proper data placement.
- Transformation: Setting rules for standardizing data values and ensuring data is appropriately formatted.
Quality governance:
- Monitoring: Setting up alerts and notifications for errors, inconsistencies, and incomplete data.
- Reconciliation: Proactively checking data for inconsistencies, missing elements and anomalies to improve overall data quality.
Data governance provides the strategic oversight and policy framework that guides these activities, ensuring data is managed consistently and in compliance with organizational standards and regulations. For more info on data governance, check out our full guide to the six building blocks of data governance here.
Data quality Vs. data governance: What's the difference?
Here’s where the distinction between the two becomes crucial. Data quality is an objective, a goal that your organization strives to achieve. Data governance, on the other hand, is the means to achieve that goal. Through a well-implemented data governance framework, you create the environment necessary to maintain and improve data quality.
To lay things out a bit more clearly, here’s a list of how data quality and governance differ in scope and focus, objective, and the activities they cover…
Scope and focus:
- Data quality: Focuses on the attributes of the data itself, ensuring it is accurate, complete, consistent, unique, timely, and valid. It deals specifically with the integrity of individual data elements.
- Data governance: Encompasses a broader strategy for managing data across the organization, including policies, roles, and procedures for overall data management. It addresses how data is accessed, secured, and maintained throughout its lifecycle.
Objective:
- Data quality: Aims to ensure that data is reliable and actionable, meeting specific standards for accuracy and completeness to support effective decision-making.
- Data governance: Seeks to establish a structured framework to manage data efficiently and securely, ensuring compliance with policies and regulations while supporting business goals.
Activities involved:
- Data quality: Involves activities such as data cleansing, validation, quality assessments, and monitoring metrics to maintain data integrity.
- Data governance: Includes developing data management policies, defining roles and responsibilities, implementing data access controls, and ensuring regulatory compliance.
Why are data quality and data governance important for marketers?
Effective data management is not just about having good data or robust policies — it's about ensuring that these two elements work seamlessly together. Data quality and data governance are two fundamental aspects that must be integrated to form a solid foundation for any data-driven organization. Here are some of the key benefits that marketers can expect to see from excellent data quality and data governance.
Data quality ensures that the data collected and used is accurate, complete, consistent, unique, timely, and validated. This level of quality allows organizations to:
-
- Make informed decisions with confidence.
- Enhance operational efficiency by reducing errors and rework.
- Improve customer satisfaction by delivering accurate and relevant interactions.
- Drive strategic initiatives based on reliable insights.
-
- Protect sensitive data and ensure compliance with relevant regulations.
- Establish clear accountability for data management tasks.
- Facilitate easier access to data for users who need it, enhancing productivity and innovation.
- Manage and mitigate risks associated with data handling and storage.
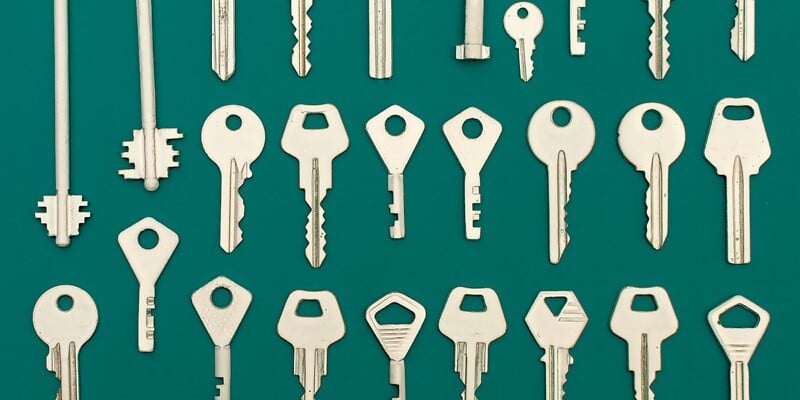
Together, high data quality and effective data governance prevent the common pitfalls of data management, such as data breaches, compliance issues, and poor decision-making based on faulty data. They are not just isolated objectives or processes; they are interdependent aspects that feed into each other to create a reliable and actionable data foundation.
The risks of poor data quality and data governance
Neglecting data governance and data quality can expose an organization to significant risks that may compromise its operations, reputation, and legal standing. Here are some of the critical risks associated with poor data management:
- Operational inefficiencies: Errors and redundancies increase operational costs and waste resources.
- Regulatory penalties: Non-compliance with regulations like GDPR, HIPAA, or CCPA can result in fines and legal consequences.
- Poor decision-making: Inaccurate data can mislead decision-making, potentially causing significant strategic missteps.
- Security vulnerabilities: Weak data governance may expose sensitive data to breaches, risking security incidents and loss of trust.
- Loss of customer trust: Mishandled or inaccurate data erodes customer confidence, potentially leading to attrition and a tarnished brand image.
Neglecting data governance and quality can lead to serious issues, from increased costs and regulatory penalties to poor decision-making and security risks. It’s essential to get these right to avoid significant problems down the line.
How Vodafone improved its data quality by 80%
As one of the world’s leading telecommunications providers, it will come as no surprise that Vodafone Germany has an incredibly mature online marketing strategy involving 20 different channels and more than 150 marketing campaigns annually.
However, although their marketing performance was strong and successful, their marketing data management and analysis led to frustrations and inefficiencies across the business.
Much of their data integration was being completed manually, and some sources weren’t easy to integrate - leading to data silos, a lack of visibility and collaboration across the team, and no consistent data quality control measures.
Vodafone decided it was time to act and chose Adverity as their data integration platform for “Project Neuron” - a Neutral, User-friendly, Real-time measurement Online Platform to evaluate and analyze marketing activity.
Adverity enabled the real-time integration of Vodafone’s 20 marketing sources into a centralized data lake, eliminating the data silos and providing the marketing team with a single source of truth, fully automating error detection and identification of anomalies.
Project Neuron was a huge success, with a 20% reduction in time spent on data acquisition and an increase of 80% in data quality. For more on this, you can read the full case study here.
Conclusion: Building a better data strategy
In the end, data quality and data governance are two sides of the same coin. You can’t have reliable, high-quality data without a strong governance framework to back it up. And without focusing on data quality, even the most well-crafted governance strategies will fall flat. Together, they ensure that your data isn’t just a collection of numbers and facts, but a powerful tool that drives your business forward.
So, as you evaluate your organization’s data strategy, ask yourself: Do you have the right governance in place to support the quality of your data? And is your data quality up to the standards needed to fuel your business decisions? Balancing both will help you not just manage your data, but truly harness its potential.