In the ever-evolving world of data analysis, imagine your data as travelers on a long journey. Sometimes, a few of these travelers don't arrive on time, and this delay can cause chaos in your data analysis.
This is where the concept of data completeness comes into play. In this blog post, we'll dive into the significance of having complete data, explore the reasons behind data gaps, and discover how data completeness checks act as gatekeepers within the validation process to ensure everything arrives intact and accurate.
What is data completeness?
Data completeness is a measure of whether all the puzzle pieces of data from different data sources have reached their destination, creating a complete picture in your target system.
Why does data completeness matter?
Imagine if data analysts notice discrepancies between their dashboards' numbers and the original data source. This can lead to doubts and mistrust in the application's accuracy. Trust in data is the foundation for creating a culture that relies on data for decision-making.
Analysts need to be absolutely confident that the data they see is both complete and accurate. In a worst-case scenario, trusting inaccurate data might lead to decisions that do more harm than good.
What is a data completeness check in data validation?
A completeness check ensures all the necessary tasks for moving data from one point to another have been successfully completed. These tasks include:
- Extracting data using APIs from third-party platforms.
- Making sure the data is in the right format.
- Cleaning and filtering the data.
- Adding extra information to the data.
- Standardizing data from different sources to a common format.
- Storing data in a data warehouse.
- Getting the data from the warehouse to display in the target system.
Errors can crop up at any of these stages, potentially leading to an incomplete final dataset. If any step fails, data analysts can't be fully confident in the accuracy of the numbers displayed in dashboards or other applications.
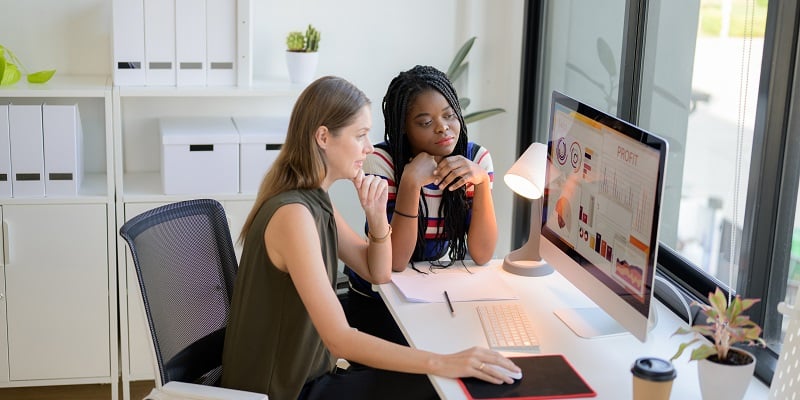
How does a data completeness check work?
Imagine a diligent assistant that automatically checks if all the tasks needed for data movement have been completed. If an error occurs, it takes note of all the important details and brings it to the right person's attention. Often, errors need human intervention to be fixed. A reliable data completeness check even suggests actionable solutions. For instance, if your data integration tool loses access to a source's authorization (e.g., Instagram), the completeness check notifies the owner of that Instagram account to re-authorize. Once re-authorized, a message is sent to the data manager to retry tasks dependent on that authorization.
Common challenges and best practices for implementing a data completeness check
If your analytics setup involves various disconnected tools, it's like managing a team of messengers who might misunderstand each other. For instance:
-
Your data sources need to talk to your ETL tool.
-
Your ETL tool has to communicate with your storage.
-
Your storage should sync with your transformation tools.
-
Your transformation tools need to interact with your visualization tools.
At each step, something could go wrong, requiring manual checks for each connection to ensure everything went smoothly. But with an integrated platform that brings all these elements together, data completeness checks are built-in and ready to use.
Methods for data completeness validation
Ensuring data completeness is a critical step in maintaining data quality. Various methods and techniques can be employed to validate data completeness effectively. In this section, we'll explore different approaches, such as statistical analysis, data profiling, and the use of data quality tools. You'll gain insights into when to use each method and how they contribute to the overall accuracy of your data.
1. Statistical Analysis
Statistical analysis is a powerful method to validate data completeness. It involves the examination of data distribution, missing values, and outliers. Statistical tests and algorithms can identify irregularities in the data. For instance, you can use summary statistics like mean, median, and standard deviation to detect missing data patterns. High variance in a particular field may indicate missing data. Statistical techniques like regression analysis can also help predict missing values based on existing data. This method is particularly useful when dealing with large datasets, as it provides a quantitative understanding of completeness.
2. Data Profiling
Data profiling involves the systematic analysis of data sources to understand their structure, content, and quality. It helps in identifying missing data by examining patterns and anomalies within the dataset. Data profiling tools can automatically generate summaries, histograms, and frequency distributions, making it easier to spot gaps in the data. Data profiling can also reveal data anomalies, such as duplicate records or inconsistent data, which may indirectly point to missing values.
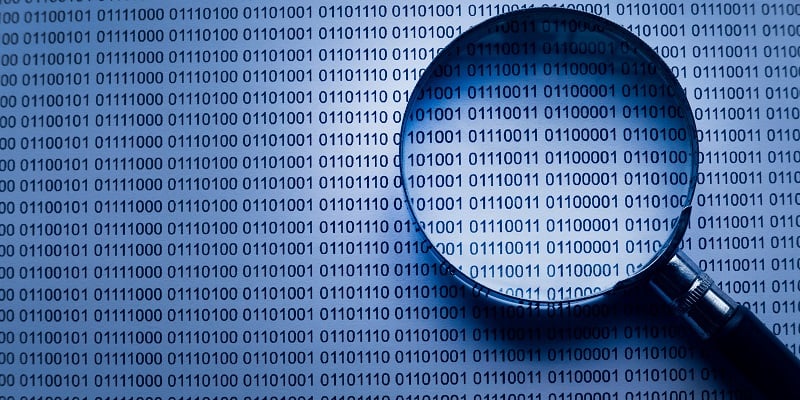
3. Data Quality Tools
Data quality tools are specialized software solutions designed to assess and improve data quality, including completeness. These tools often offer a range of functionalities, such as data profiling, data cleansing, and data enrichment. They can automatically flag missing data, provide data lineage information, and suggest ways to fill gaps, enhancing the overall quality of data. Organizations can integrate data quality tools into their data pipelines to perform ongoing completeness checks.
4. Sampling and Manual Review
In some cases, especially when dealing with small datasets or unique data sources, manual review and sampling may be necessary. Data analysts can review a subset of the data to identify any missing values or inconsistencies. While this method is more resource-intensive, it can be highly effective for specialized datasets where automated methods may not be suitable.
5. Cross-Validation
Cross-validation is a technique commonly used in machine learning and predictive modeling. It can also be applied to data completeness validation. By splitting the dataset into multiple subsets and validating each subset against the others, you can identify discrepancies and missing values. Cross-validation helps ensure that data completeness is consistent across different parts of the dataset.
Data completeness metrics
Measuring data completeness is vital for organizations aiming to make data-driven decisions. To achieve this, specific metrics and key performance indicators (KPIs) come into play. In this section, we'll delve into various data completeness metrics that organizations can utilize to gauge the quality of their data. Discover how these metrics help in monitoring data completeness over time and improving data quality as a whole.
1. Data Completeness Ratio
This metric calculates the percentage of complete records in a dataset compared to the total number of records. It's a straightforward way to measure the overall completeness of the data. A higher completeness ratio indicates a more complete dataset.
2. Missing Data Percentage
This metric quantifies the proportion of missing values in specific fields or columns. It helps pinpoint which attributes or variables are more prone to data gaps. Monitoring changes in the missing data percentage over time can indicate data quality improvements or issues.
3. Completeness by Source
Organizations often collect data from various sources. This metric assesses the completeness of data from each source individually. It allows organizations to identify which data providers or channels are more reliable in delivering complete data.
4. Completeness Over Time
Tracking data completeness over time can reveal trends and patterns. Organizations can create time-series charts to visualize how data completeness evolves and take proactive steps to address any declining trends.
5. Completeness by Data Type
Different data types (e.g., text, numeric, categorical) may have varying levels of completeness. This metric categorizes data completeness by data type, helping organizations focus their efforts on improving the completeness of critical data types.
6. Data Entry Completeness
For user-generated data, such as form submissions or customer feedback, this metric assesses the completeness of data entry. It may involve validating whether mandatory fields are consistently filled out or identifying common entry errors.
7. Data Completeness Score
Some organizations create composite scores that weigh different completeness metrics based on their importance. This score provides an overall assessment of data completeness and can be used for benchmarking and goal-setting.
8. Data Completeness Trend Analysis
In addition to static metrics, tracking the trend in data completeness over time is essential. Are completeness levels improving, deteriorating, or remaining stable? This analysis can help organizations take timely corrective actions.
By implementing these methods and metrics, organizations can systematically validate data completeness and maintain high-quality data that supports accurate decision-making and analytics.
Conclusion
Wrapping up, we've explored the critical concept of data completeness and how data completeness checks act as guardians of accurate analysis. In a world where data-driven decisions rule, understanding and implementing these checks is like having a safety net for your insights.